It’s another Tuesday, and this week, we’re diving into a concept that’s quietly powering some of the smartest AI systems today—RAG.
🔍 So, what is RAG?
Retrieval-Augmented Generation is an approach where an AI model doesn’t rely only on what it was trained on. Instead, it retrieves relevant information from external sources (like documents, databases, or websites) in real-time to generate more accurate, up-to-date, and context-aware responses.
It’s like giving your AI a research assistant.
🧠 Why is RAG Important?
Traditional language models are limited to the knowledge they were trained on, and they often “hallucinate” answers when they’re unsure. With RAG:
The AI can pull facts from external knowledge bases
It reduces hallucinations by grounding answers in real data
It enables domain-specific applications (e.g., law, finance, health) using your own content
📌 Real-World Use Cases
✅ A company chatbot that references internal HR docs to answer employee queries
✅ A healthcare AI assistant that retrieves clinical guidelines to support diagnoses
✅ A legal research assistant that references specific case laws in its answers
🚀 Why Your Organization Should Care
If your business has structured documents, PDFs, reports, or FAQs, you already have what it takes to build a custom AI assistant using RAG.
Instead of retraining models from scratch, RAG lets you plug in your knowledge and start generating smarter responses—today.
The future isn’t just generative—it’s retrieval-powered.
It will also interest you to know that I worked on a Retrieval-Augmented Generation (RAG) project for my MBA.
Next week, I’ll share insights from that experience—and introduce you to TsotsooAI.com, a real-world application of RAG in action. Stay tuned! 🚀
What questions do you have about RAG? Is your organization exploring it yet? Let’s talk in the comments.
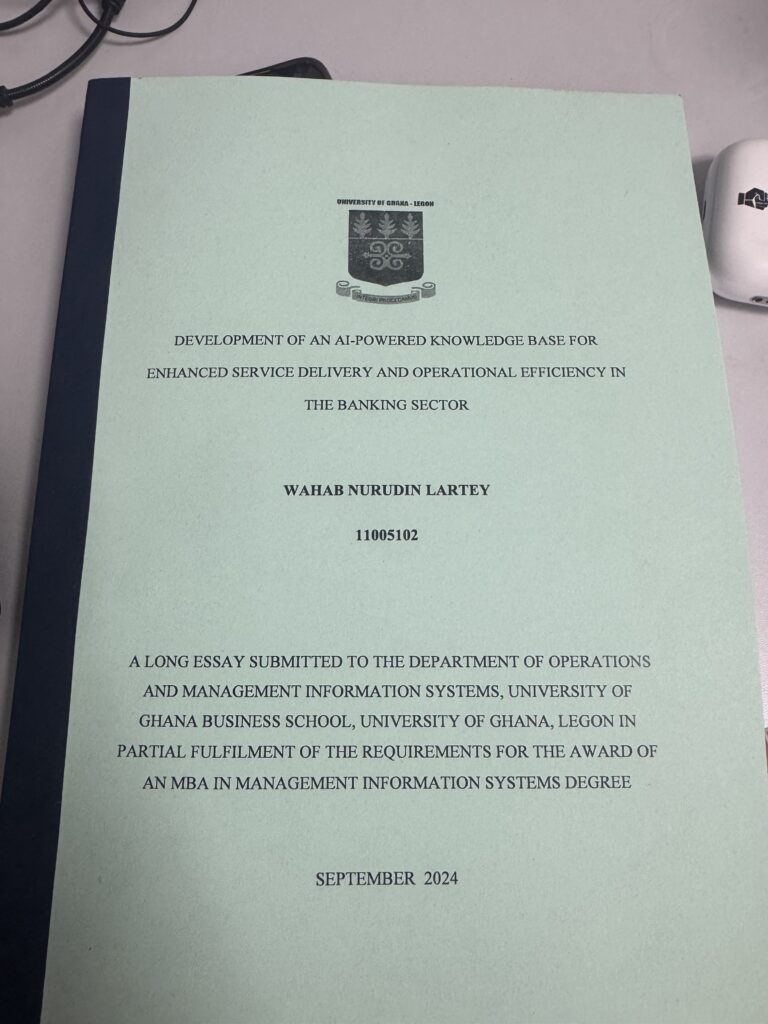